Time Series Analysis, Forecasting, and Machine Learning
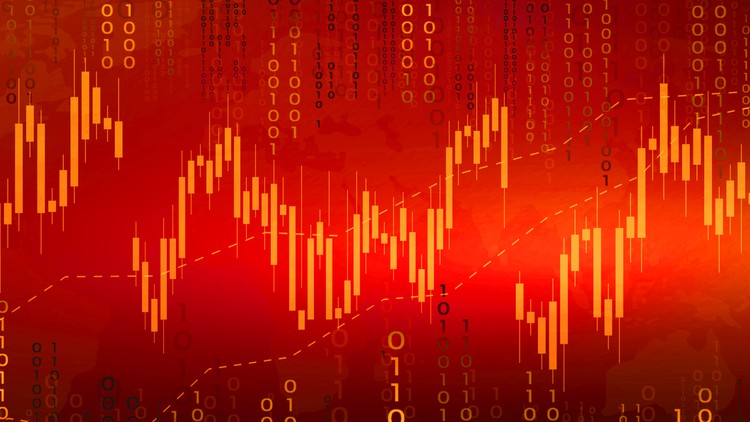
Why take this course?
🛣️ Dive into the World of Time Series Analysis with Python!
Welcome to Mastering Time Series Analysis, Forecasting, and Machine Learning with Python!
Time Series Analysis has rapidly ascended in significance in our data-driven world. 🌍 From navigating economic uncertainties 💰 to managing public health responses, 🤫 to optimizing business operations, 🏢 the ability to forecast and analyze time series data is more critical than ever.
Course at a Glance:
This isn't just another Time Series Analysis course. We're on the cutting edge of machine learning and statistics, incorporating modern techniques that are shaping the future of predictions and analytics. Here's what you can expect:
-
Advanced Techniques: Dive deep into state-of-the-art methods including Deep Learning, Time Series Classification, and more. 🧠✨
-
Comprehensive Coverage: Master a wide range of models from classical statistic approaches like ARIMA to the latest machine learning and deep learning models. 📊
-
Real-World Applications: Learn through practical applications such as forecasting sales data, stock market trends, and interpreting smartphone usage patterns. 📈💼📱
What You'll Learn:
-
ETS & Exponential Smoothing: Understand exponential smoothing techniques to handle time series with trend and seasonal components.
-
Holt's Linear Trend Model: Discover how to apply Holt's linear trend model for more accurate predictions.
-
Holt-Winters Model: Learn the power of the Holt-Winters method for forecasting with trend and seasonality.
-
ARIMA, SARIMA, SARIMAX & Auto ARIMA: Master Advanced Regression Integrated Moving Average (ARIMA) models, their variants, and how to automate the model selection process.
-
ACF & PACF: Explore Autocorrelation Function (ACF) and Partial Autocorrelation Function (PACF) for diagnostic checking of time series models.
-
Vector Autoregression & Moving Average Models (VAR, VMA, VARMA): Understand vector time series modeling and the importance of VARMA models.
-
Machine Learning Models: Explore Logistic Regression, Support Vector Machines, Random Forests, and more to forecast categorical data.
-
Deep Learning Models: Uncover the secrets behind Artificial Neural Networks (ANNs), Convolutional Neural Networks (CNNs), Recurrent Neural Networks (RNNs), GRUs, and LSTMs in the context of time series forecasting.
-
Forecasting with AWS & Prophet: Learn to use Amazon's AWS Forecast service and Facebook's FB Prophet for real-world applications.
-
GARCH: Dive into Generalized Autoregressive Conditional Heteroskedasticity (GARCH) for modeling financial time series volatility.
Exclusive VIP Features:
-
Detailed Code Analysis: Every line of code is meticulously explained, and you're encouraged to challenge any assumptions through direct email communication with the instructors. 🤝
-
No Time Wasted: Say goodbye to fluff; this course avoids unnecessary "typing" time during lessons, ensuring every minute is valuable. ⏳
-
University-Level Math: Engage with algorithms at a deeper level, touching on aspects often omitted by other courses. 🎓
Why Choose This Course?
This course stands out because it offers:
-
Lifetime Access: Join us today and have access to the course materials for life! 🗝️
-
Certificate of Completion: Show off your new skills with a certificate you can proudly add to your LinkedIn profile. 🏆
-
Learn Cutting-Edge Techniques: Gain expertise in the latest time series analysis techniques that you won't find anywhere else. 🚀
Don't miss out on this opportunity to revolutionize your data analysis and forecasting capabilities with Python. Enroll now and take your first step towards becoming a master in Time Series Analysis, Forecasting, and Machine Learning. We look forward to welcoming you into the course and on this transformative learning journey! 🌟🐍
Sign up today and unlock the power of data forecasting with Python!
Course Gallery
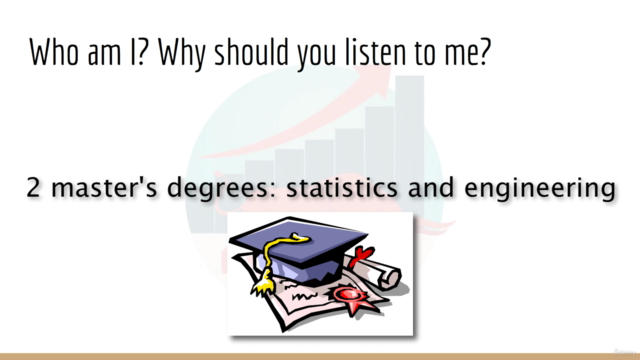
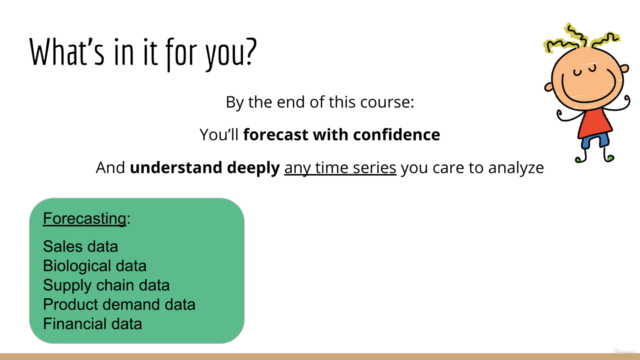
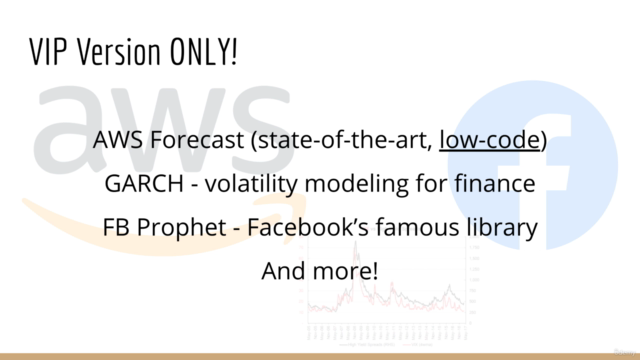
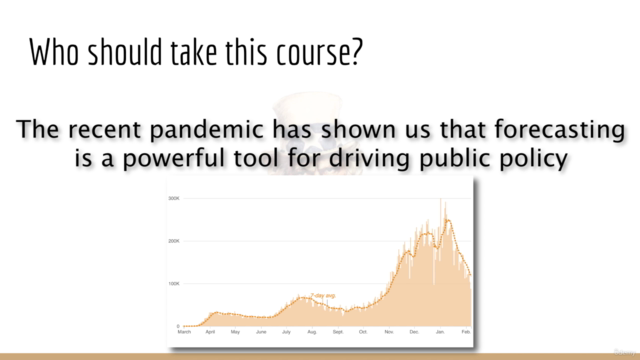
Loading charts...
Comidoc Review
Our Verdict
This 23.5 hour Time Series Analysis and Forecasting course on Udemy is led by an experienced and knowledgeable instructor who provides a thorough examination of various time-series analysis techniques—from exponential smoothing and ARIMA to machine learning and deep learning models. While some students might find specific mathematical concepts challenging without adequate prior exposure or intuitive understanding, the overall curriculum offers valuable insights and practical applications for data professionals eager to augment their time-series forecasting capabilities.
What We Liked
- Comprehensive coverage of time series analysis techniques, including ETS, Holt's Linear Trend Model, ARIMA, GARCH, and deep learning methods
- High-quality course materials, including videos and notebooks, providing a hands-on learning experience
- Excellent communication skills of the instructor, explaining complex concepts clearly and breaking down complex topics for better understanding
- Well-rounded curriculum with a strong emphasis on non-deep learning statistical models for time-series prediction
Potential Drawbacks
- Some students may find it challenging to follow certain mathematical concepts without prior exposure or intuitive understanding, such as CNNs and GARCH
- Lack of slides provided along with the videos might be inconvenient for some learners who prefer written summaries of the content
- Limited focus on error or anomaly detection outside of Facebook Prophet compared to other time-series models
- A small portion of students may find the instructor's approach condescending, potentially affecting their overall experience