Python & Machine Learning for Financial Analysis
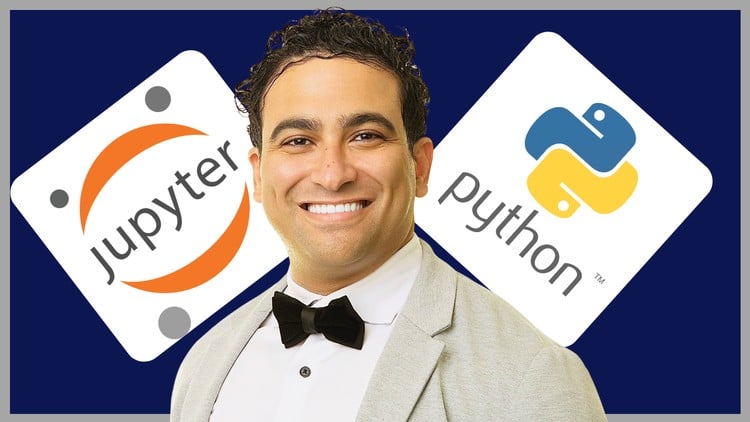
Why take this course?
🎓 Master Python Programming Fundamentals and Harness the Power of ML for Financial Analysis 🚀
Course Headline:
The Complete Python and Machine Learning for Financial Analysis
Course Description:
Are you ready to dive into the world of Python programming and apply it to solve real-world financial analysis problems? If your answer is a resounding "yes," then you've come to the right place! 🎉
Welcome to the comprehensive "Python & Machine Learning for Financial Analysis" course! Here, you'll learn everything you need to tackle practical finance and banking applications using Python. This isn't just theoretical knowledge—you'll be applying what you learn directly to real-world scenarios in the financial sector.
Why Choose Python? 🐍
Python is the reigning champion of programming languages, especially when it comes to AI & Machine Learning (ML). Here's why you should consider learning Python right now:
- #1 Language for AI & ML: Python leads the pack in machine learning and artificial intelligence applications.
- Easy to Learn: Python is accessible, even for those new to coding.
- High Demand: Python developers are in high demand, with a low supply—making it an ideal skill to learn.
- High Salary: The average salary for Python programmers in the US is around $116 thousand dollars annually!
- Scalability: Python's power and scalability make it the backbone of giants like Google, Instagram, YouTube, and Spotify.
- Versatility: From data science to web development, gaming, robotics, and beyond—Python is the jack-of-all-trades in programming.
Course Unique Features:
This course is meticulously structured into three main parts, ensuring a comprehensive understanding of each subject area:
-
Part #1 – Python Programming Fundamentals:
- Essentials like data types, variables, loops, conditionals, functions, and file operations.
- Key libraries for data science such as Numpy and Pandas.
- Data visualization tools including Matplotlib, Seaborn, Plotly, and Bokeh.
-
Part #2 – Financial Analysis in Python:
- Insights into financial concepts like portfolio returns, risk analysis, Sharpe ratio, and trading strategies.
- Exploration of the Capital Asset Pricing Model (CAPM), Markowitz portfolio optimization, and efficient frontier.
-
Part #3 – AI/ML in Finance/Banking:
- Real-world projects applying Deep Neural Networks (LSTMs) for stock price predictions.
- Unsupervised machine learning strategies like K-Means Clustering and Principal Components Analysis for customer segmentation.
- Basics of Natural Language Processing (NLP) to analyze stock sentiments.
Learning Approach:
This course is designed with a hands-on, project-based approach:
- Practical Application: With mini challenges and exercises throughout the course, you'll learn by doing.
- Real-World Experience: More than 6 practical projects to build your portfolio.
- Access to Materials: All codes and slides are provided for your convenience.
- Professional Recognition: Earn a certificate of completion to showcase on LinkedIn.
- Risk-Free Trial: A 30-day money-back guarantee lets you try the course risk-free!
Who Should Enroll?
This course is ideal for:
- Financial professionals looking to leverage data science in their roles.
- Python beginners and data scientists eager to expand their skills into finance.
- Investment bankers and financial analysts aiming to advance their careers through practical experience.
No prior Python or programming experience is required! If you're a complete novice, clear video explanations will guide you from the basics onward.
Join us today and embark on a transformative learning journey with "Python & Machine Learning for Financial Analysis" 💡✨
Ready to Get Started?
Enroll now and let's turn your curiosity into expertise! With this course, you'll not only gain valuable skills but also open doors to career advancement and exciting opportunities in the world of finance and data science. 🚀🌟
Course Gallery
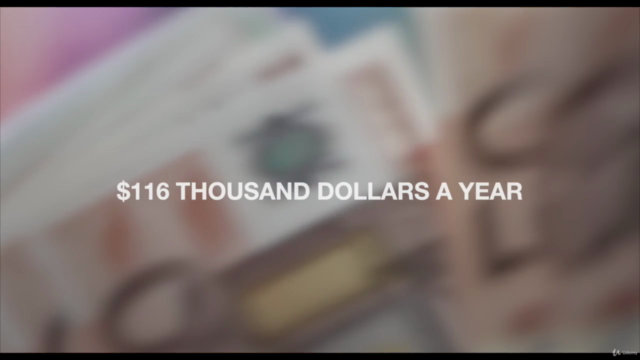
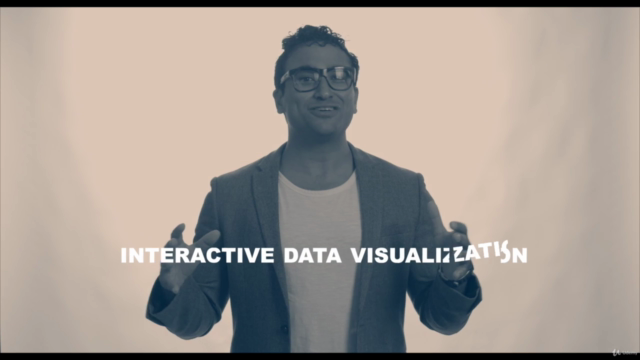
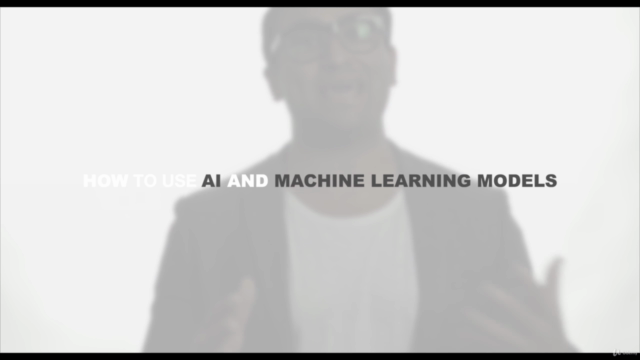
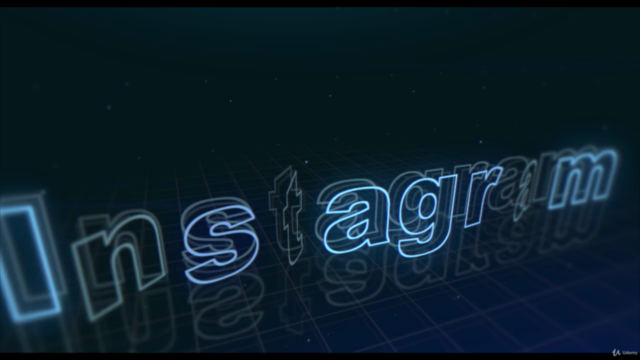
Loading charts...
Comidoc Review
Our Verdict
This course effectively combines Python programming, data science, and machine learning, specifically tailored to financial applications. While it does provide essential building blocks for beginners, it might not be an ideal entry point for those entirely new to the subject matter due to some inconsistencies in pacing and depth of explanation. Despite a few areas that require improvement, its strong focus on practical problem-solving sets it apart from other courses.
What We Liked
- Comprehensive coverage of Python programming fundamentals for data science and machine learning, specifically applied to finance.
- Detailed explanations of key financial concepts such as calculating daily portfolio returns, risk and Sharpe ratio.
- In-depth exploration of the theory and intuition behind various machine learning algorithms for regression, classification and clustering, with practical applications in finance.
- Hands-on experience with popular Python libraries like NumPy, Pandas, Matplotlib/Seaborn, and SciKit-Learn.
Potential Drawbacks
- Some students expressed disappointment with the AI/ML section, citing insufficient explanation of function parameters and lack of a comprehensive introduction to some concepts.
- Certain sections seem rushed with abrupt transitions, causing confusion for learners unfamiliar with the subject matter.
- Assessment of machine learning models' performance can be more thoroughly explained, especially regarding real-world applicability.
- Lack of clarity in feature engineering and data cleaning processes.