Data Science: Supervised Machine Learning in Python
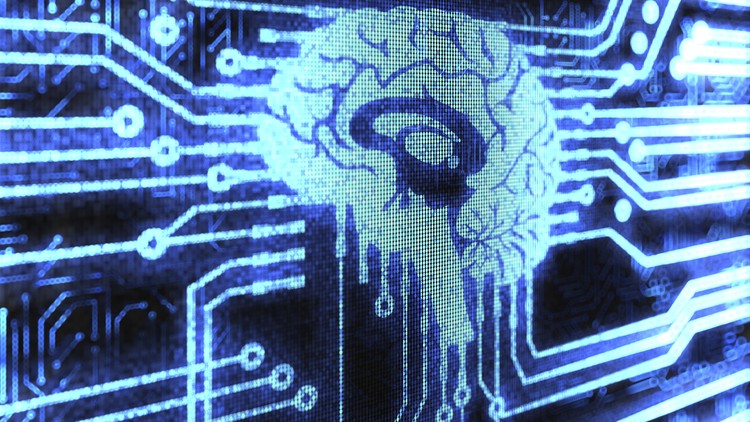
Why take this course?
🌟 Master Data Science with Python: Supervised Machine Learning Algorithms 🌟
Course Overview:
Machine learning has revolutionized the way we approach problems, from diagnosing diseases to autonomous driving. It's at the heart of innovation and is reshaping industries across the globe. Google's "machine learning first" philosophy exemplifies the growing importance of machine learning in our tech-driven world. This course dives deep into implementing classic machine learning algorithms with a focus on understanding, not just using them.
🚀 What You'll Learn:
- 🔍 K-Nearest Neighbor (KNN): We'll kick off by exploring this intuitive algorithm, understanding its strengths and weaknesses, and implementing it from scratch.
- 🎲 Naive Bayes & General Bayes Classifier: These probabilistic gems will reveal the magic of Bayesian inference and how they can be transformed into efficient models.
- 🌳 Decision Trees: We'll tackle the complexity of decision trees, a key algorithm that's often overlooked in other courses, and learn by implementing them ourselves.
- 🤖 Perceptron & Neural Networks: Trace the lineage of perceptrons to modern neural networks and deep learning, understanding their place in machine learning.
🛠️ Practical Skills:
- Mastering hyperparameters, cross-validation, feature extraction, and feature selection.
- Comparing machine learning approaches with deep learning.
- Utilizing the powerful Sci-Kit Learn library for real-world applications.
- Building a web service that deploys a machine learning model for making predictions.
💻 Hands-On Learning:
This course is designed to give you a deep understanding of machine learning algorithms by implementing them from scratch. You'll learn to visualize what's happening internally within these models, ensuring you truly grasp the concepts.
Course Requirements:
- A basic understanding of Python programming (if/else, loops, lists, dicts, sets).
- Familiarity with calculus and probability theory is beneficial for a deeper dive into certain aspects of machine learning.
- Prior knowledge of Numpy and Scipy will be advantageous.
- A curiosity for learning and a passion for uncovering the secrets behind algorithms.
Order Your Learning Journey:
For newcomers and seasoned learners alike, check out the "Machine Learning and AI Prerequisite Roadmap" in any of my courses, including the free Numpy course, to determine the best sequence to take these courses.
🎓 Unique Features:
- Every line of code is thoroughly explained to ensure you understand the inner workings of algorithms.
- No filler content; every minute is dedicated to learning through meaningful examples.
- We embrace advanced mathematics, ensuring no important details are left out.
Join us on this journey to demystify machine learning and turn your passion for coding into mastery over data science. With hands-on experience and a focus on true understanding, you'll be well-equipped to tackle any data-driven challenge that comes your way! 🚀📚💫
Prerequisites:
- Basic Python programming skills (if/else, loops, lists, dictionaries, sets)
- Comfortable with calculus concepts (not strictly required but highly recommended for a deeper grasp of the algorithms)
- Familiarity with probability theory (continuous and discrete distributions, joint, marginal, conditional, PDF, PMF, CDF, Bayes rule)
- Basic understanding of Numpy and Scipy is beneficial
Learning Path:
Before you embark on this course, it's important to understand the sequence in which you should take these lessons. Refer to the "Machine Learning and AI Prerequisite Roadmap" for guidance on optimizing your learning path. This roadmap is available in the FAQ section of any of my courses, including the free Numpy course.
Elevate your data science skills with hands-on machine learning implementation, deep insights, and a curriculum that goes beyond mere coding. With Lazy Programmer's unique approach to teaching, you'll not only learn machine learning algorithms but also appreciate their intricacies and capabilities. Join the ranks of those who truly understand machine learning by implementing it from scratch! 🤓👨💻🧠
Course Gallery
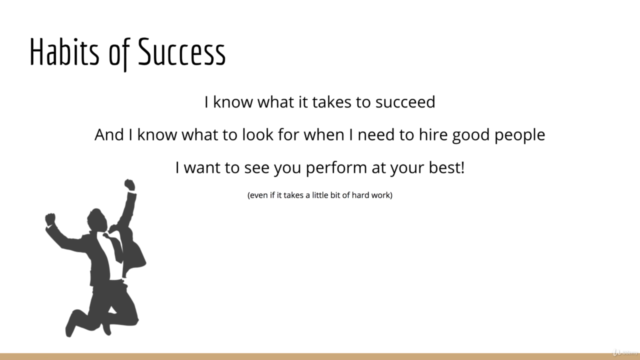
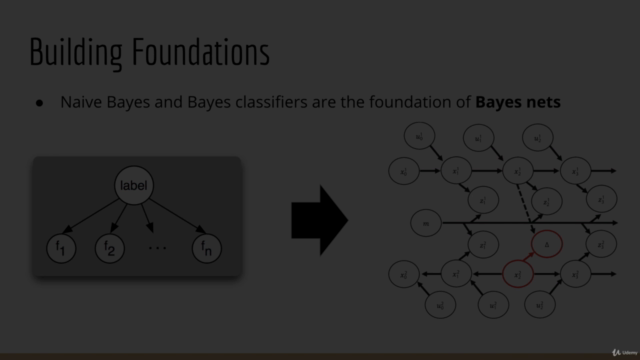
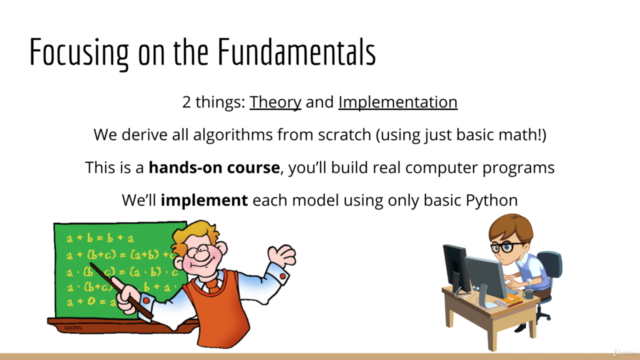
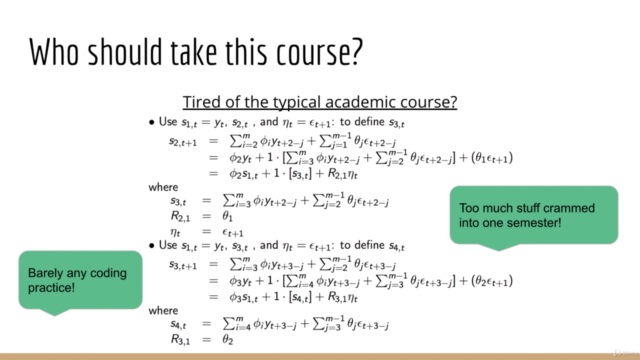
Loading charts...
Comidoc Review
Our Verdict
This course excels in providing hands-on experience with classic machine learning algorithms while incorporating essential theory and intuition. However, some students may find content lacking or certain topics moving too quickly without properly explaining statistical concepts. As such, this course best serves those with prior knowledge looking to advance their understanding of these Python-based models.
What We Liked
- Covers the implementation of several classic machine learning algorithms in Python and with Scikit-Learn
- Clear explanations of theory related to K-Nearest Neighbors, Naive Bayes, Decision Trees, and Perceptron
- Code implementations made readily available and approachable for learners
- Focus on understanding the intuition behind these algorithms instead of just coding them
Potential Drawbacks
- Some reviewers mention feeling that some content is missing or not covered in sufficient depth, such as regression cases for Decision Trees or tree pruning
- Concerns about pacing and assuming prior statistical knowledge
- Implementations of algorithms mentioned to be slower than alternative solutions
- Minimal coverage on building a machine learning web service compared to expectations